A U.S.-Dutch research team has developed a novel bidding strategy for PV plant operators participating in electricity spot markets. It is based on turning probabilistic solar power forecasts into interdependent scenarios used in the multistage bedding strategy.
According to the researchers, the new method is able to provide increased revenues and reduced imbalances. “Accurate forecasts can assist in the timely scheduling of the dispatch of power generators and batteries, and therewith ensuring grid stability, while reducing the need for balancing reserves,” they said. “There is a lack of studies that develop and evaluate operational stochastic bidding strategies for electricity markets that consider PV systems and rely on probabilistic solar power forecasting models.”
The bidding strategy is designed to optimize bidding results, considering the uncertainty of PV power generation. It first aims to maximize revenues from the day-ahead market (DAM) by considering a set of scenarios, which are a range of possible power outputs. In order to achieve this goal, it used the Pinson method, a statistical technique that can transform probabilistic forecasts to scenarios that consider the interdependence structure of the prediction errors.
“The method was originally developed for the purpose of wind power forecasting and was later also successfully used for net load forecasting, i.e. demand subtracted with solar generation,” the academics explained.
Following the creation of several scenarios, a bid is generated by solving a few numerical problems. Then, corrections to the initial DAM bid are made in the intraday market (IM), using updated PV power forecasts. “The consequences of deviations in real-time are considered by the imbalance prices for up- and down-regulation,” added the team.
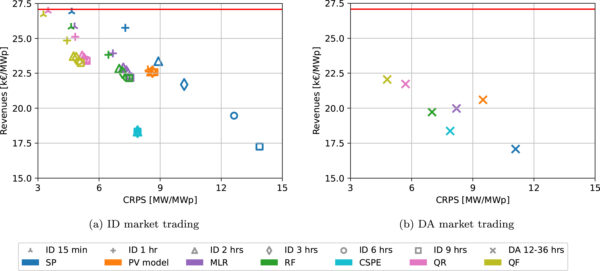
The scenarios made with the Pinson method were based on three probabilistic models – quantile regression (QR), quantile regression forest (QF) and clear sky persistence ensemble (CSPE). They were all benchmarked against point prediction methods, that provide a single predicted value for each period.
“The point forecasting models include a multi-variate linear regression (MLR), random forest regression (RF), physical PV and a smart (clear sky) persistence (SP) model,” the scientists explained. “Since these point forecasts do not provide any information regarding the uncertainty of the prediction, the potential monetary impact of an imbalance is not considered.”
All prediction mechanisms were then run on data manufactured from a simulation of a 1 MW system in Cabauw, the Netherlands. The analysis was based on real-measured global horizontal irradiance (GHI), ambient and dew point temperature, wind speed, and surface pressure from 2018 to 2020. The first two years of collected data were used to train the different prediction models, while 2020 was used for testing.
“Results show that the probabilistic forecasting models outperform the point models,” the scientists stressed. “Second, the results demonstrate the dominance of tree-based models, where the RF and QF models outperform all other DA and ID point and probabilistic models, respectively. The findings indicate that the proposed method outperforms the reference method, yielding higher revenues and causing less imbalance.”
Also, the analysis showed that expanding market participation from the DAM to the IM results in increased revenues. “Considering the best-performing model per forecast horizon, revenues increase with 22.3% from € 22,000 ($ 23,900) /MW to € 27,000 /MW per year when DAM trades are updated with IM trades,” they concluded. “Similarly, the imbalance caused almost halves, by -46.8%.”
Their findings were presented in “Probabilistic solar power forecasting: An economic and technical evaluation of an optimal market bidding strategy,” published on Applied Energy. The group included researchers from Utrecht University, Wageningen University, and the University of California, San Diego.
This content is protected by copyright and may not be reused. If you want to cooperate with us and would like to reuse some of our content, please contact: editors@pv-magazine.com.
By submitting this form you agree to pv magazine using your data for the purposes of publishing your comment.
Your personal data will only be disclosed or otherwise transmitted to third parties for the purposes of spam filtering or if this is necessary for technical maintenance of the website. Any other transfer to third parties will not take place unless this is justified on the basis of applicable data protection regulations or if pv magazine is legally obliged to do so.
You may revoke this consent at any time with effect for the future, in which case your personal data will be deleted immediately. Otherwise, your data will be deleted if pv magazine has processed your request or the purpose of data storage is fulfilled.
Further information on data privacy can be found in our Data Protection Policy.